Probably Approximate Safe RL Policy
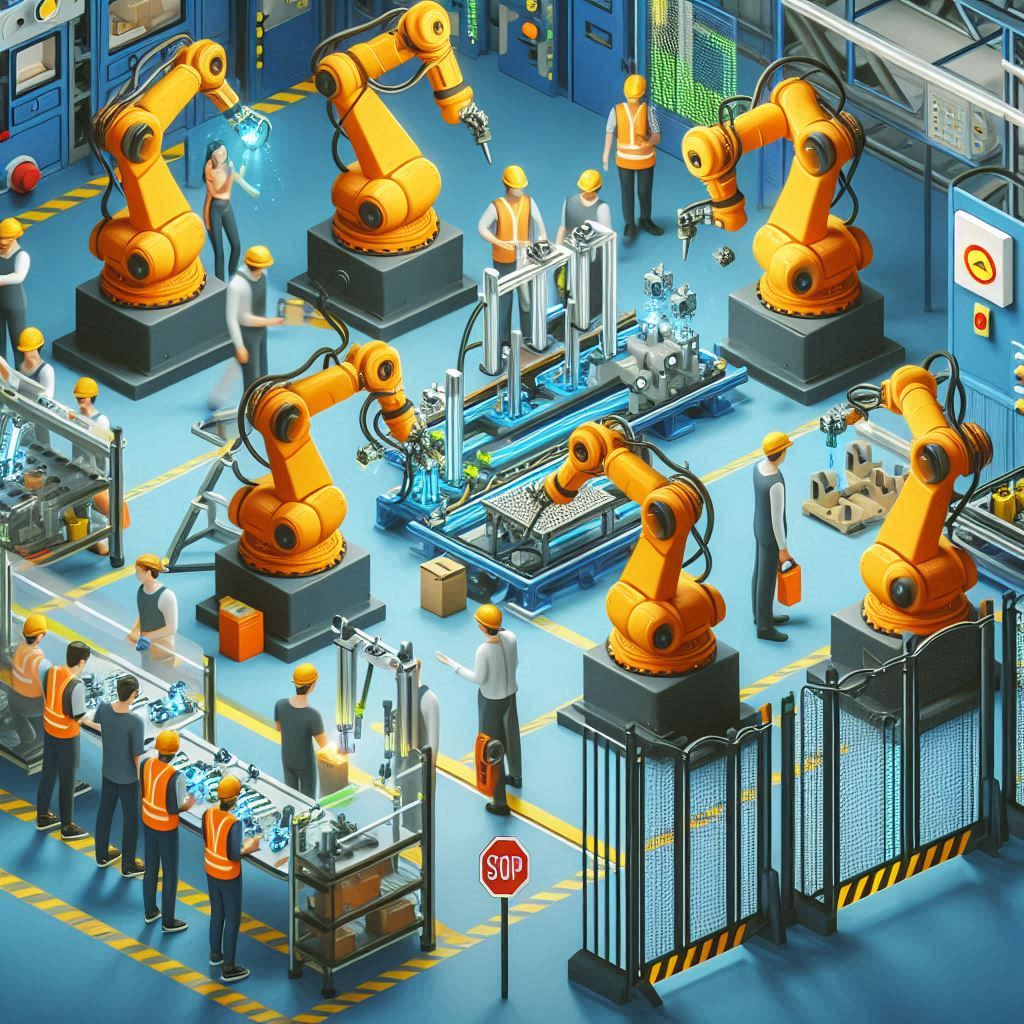
With the advancement of machine learning based automation in the current digital world, the problem of safety verification of such systems is becoming crucial, especially in safety-critical domains like self-driving cars, robotics, etc. Reinforcement learning (RL) is an emerging machine learning technique with many applications, including in safety-critical domains. The classical safety verification approach of making a binary decision on determining whether a system is safe or unsafe is particularly challenging for an RL system. Such an approach generally requires prior knowledge about the system, e.g., the transition model of the system, the set of unsafe states in the environment, etc., which are typically unavailable in a standard RL setting. Instead, this paper addresses the safety verification problem from a quantitative safety perspective, i.e., we quantify the safe behavior of the policy in terms of probability. We formulate the safety verification problem as a chance-constrained optimization using the technique of barrier certificate. We then use a sampling based approach called scenario optimization to solve the chance-constrained problem, which gives the desired probabilistic guarantee on the safe behavior of the policy. Our extensive empirical evaluation shows the validity and robustness of our approach in three RL domains.
[Paper: AAMAS-2024]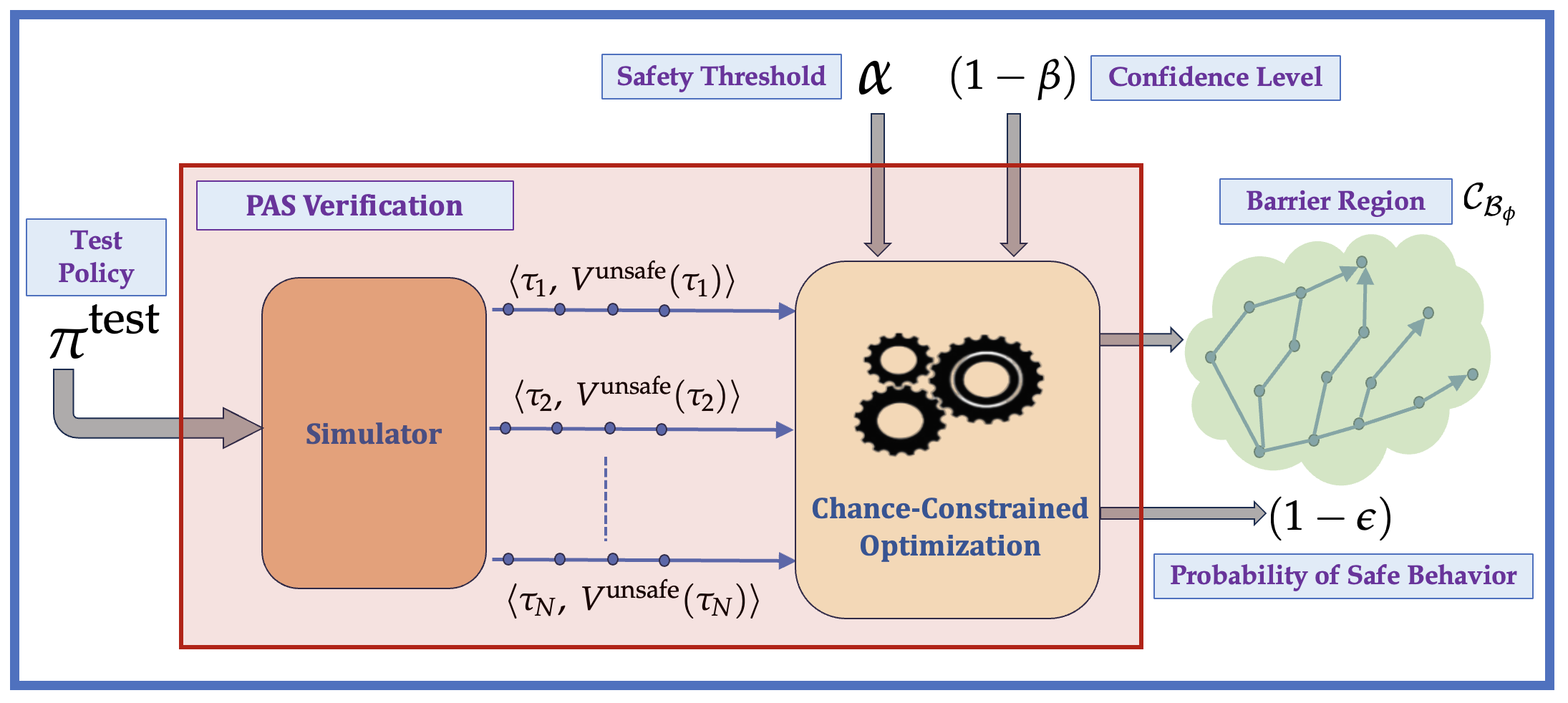
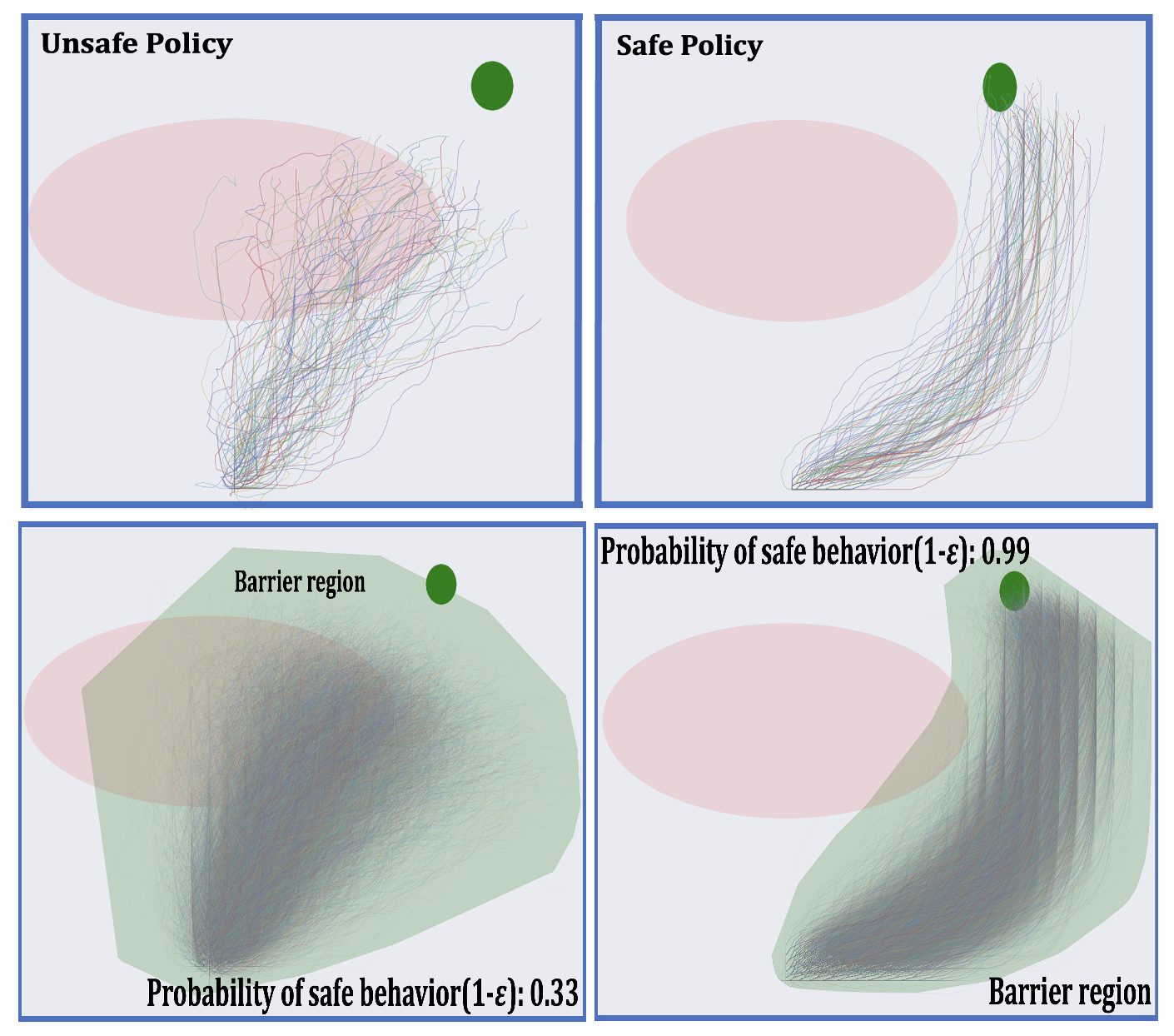